Saving breasts might be the last thing you would think a computer would have on its mind. However, saving breasts from unneeded surgery has become the machine learning mission of some medical research computers. Dartmouth researchers were led by Saeed Hassanpour, Ph.D. They developed machine learning to empower computers to “predict the likelihood that a high-risk ADH breast lesion is cancerous.”
The Backstory on Machine Learning
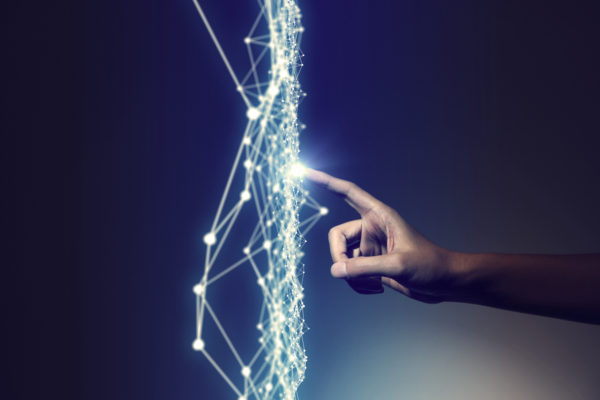
Saving Breasts from unneeded surgery can be a matter for machine learning and AI to solve.
But before explaining how it might be saving breasts, let’s review the backstory of machine learning. Machine learning is, at its heart, “the process of granting a machine or model access to data and letting it learn for itself.”
Machine Learning and Artificial Intelligence have been hot topics in the mainstream news media for months. Anyone who has ever said, “Seri, call my husband,” has experienced machine learning. Likewise, when we get advice about buying products on Amazon, it is the power of machine learning that advises us on which product will best fit our needs.
Machine Learning Meets Medical Research.
It is, of course, common knowledge that a computer can access much more data much faster than a human mind. And machine learning based special programs give the machine instructions. Piles of data, and then minimum human interference as the computer (or machine) learns what to search out, find or solve. Thus, it should be almost obvious that medical research is one of the areas which will most benefit from machine learning. For more about machine learning in medical applications, check out this informative online resource. Meanwhile, read on to see how Dr. Hassanpour’s team is using machine learning to save breasts from unnecessary surgeries.
Saving Breasts with a New Method of Machine Learning: Sparing You Needless Breast Surgery
Dartmouth researchers led by Saeed Hassanpour, Ph.D., found a very specialized machine learning method for their computers to analyze data. They were empowered to identify certain types of breast lesions and to decide if those certain ADH breast lesions are malignant. Read on to discover the importance of this.
Saving Breasts: The Research and the Results
In the tests, the machine learning strategy identified 98% of all malignant ADH breast Lesion cases prior to surgery. Thus, with Dr. Hassanpour’s machine learning programming in the computers, 16% of women with the condition can be prevented “from undergoing an unnecessary operation for a benign lesion.” And thus we have coined the expression, saving breasts.
Sparing Surgery and Saving Breasts
Thus, the “New machine learning method could spare some women from unnecessary breast surgery…” Once larger studies confirm these statistics and this conclusion, then low-risk patients and their doctors can share the options for types of treatment.
The machine learning program can help them “to decide whether active surveillance and hormonal therapy is a reasonable alternative to surgical excision.”
Let’s Take a Deeper Look at Breast Lesions
Altiora Plastic Surgery and Medspa want you to know a few facts concerning this research, in case you or someone you love is diagnosed with “Atypical ductal hyperplasia (ADH).”
- First of all, typically “ADH is a breast lesion associated with a four- to five-fold increase in the risk of breast cancer.”
Case Viewing: Fast, smart Machine Learning could be saving breasts from unnecessary surgery due to ADH.
- In the second place, doctors find ADH by using mammography.
- Doctors must identify the condition with a core needle biopsy.
- Keep in mind that in spite of several “passes of the lesion during biopsy, only portions of the lesions are sampled.”
- Unfortunately, that and other variable factors might influence the sampling. Thus “the presence of cancer may be underestimated by 10-45%.”
- The current surgical recommendation is the removal of the tissue for all ADH cases found on core needle biopsies. Statistically, “About 20-30% of ADH cases are upgraded to cancer after surgical excision.”
- Here’s our most crucial point: However, this means that 70-80% of women with ADH “undergo a costly and invasive surgical procedure for a benign (but high-risk) lesion.”
How Machine Learning is Saving Breasts
Dr. Hannapour stated, “Our results suggest there are robust clinical differences between women at low versus high risk for ADH.” And he added…” the computer decisions can upgrade from high risk for ADH to actual cancer. It bases decisions on core needle biopsy data “that allowed our machine learning model to reliably predict malignancy upgrades in our dataset,”
A Great Side Effect of the Research: More Knowledge of the Variables between High Risk and Cancer
“This study also identified important clinical variables involved in ADH upgrade risk.” (If we know the variables in the first place, we are better equipped to review their results.) Thus, Dr. Hassanpour has published, Prediction of Atypical Ductal Hyperplasia Upgrades through a Machine Learning Approach to Reduce Unnecessary Surgical Excisions.
Defeating the Long Standing Status Quo: Excision
So the long-standing treatment for these lesions has been to utilize surgery. Now machine learning is giving us new alternatives. It’s like having newer and better tests before applying a scalpel to the situation. Thus, it’s about saving breasts. Dr. Hassanpour states, “Our model can potentially help patients and clinicians choose an alternative management approach in low-risk cases.”
With machine learning in place, patients and doctors have “the ability to choose between surgical excisions for certainty versus surveillance…”
Finding New Ways of Saving Breasts with Machine Learning

Unnecessary Surgery Avoided and Summer Fun Goes on.
You see with surveillance as an option, doctors give patients the opportunity “to avoid cost, stress, and potential side effects in women at low risk of their ADH upgrading to cancer.” And Machine Learning could make this possible. But Dr. Hannapour knows that more studies are necessary on larger datasets. They are continuing to validate their approach on large external datasets using state and national breast cancer registries.
Likewise, they are collaborating with other medical centers in utilizing this new approach to saving breasts from unnecessary surgical procedures.
Additionally, the team soon hopes to enlarge their study with machine learning programs. They will include other high-risk breast lesions such as lobular neoplasia, papillomas, and radial scars. In time, we might owe machine learning a great debt for saving breasts and women’s lives.